Naive Bayes model
- 网络朴素贝叶斯模型
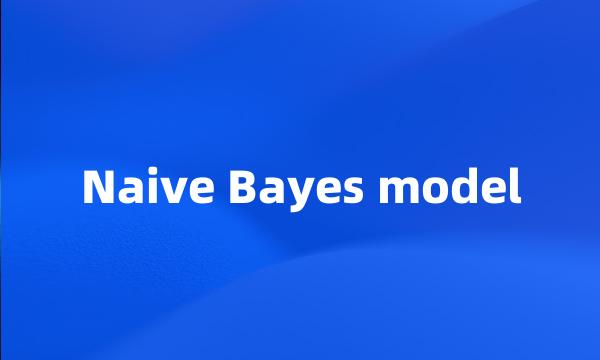
-
Compared with the logistic regression model , it suggested that the naive Bayes model showed an obvious advantage in resolution .
通过与Logistic回归模型的对比,表明朴素贝叶斯模型在分辨度方面存在明显优势。
-
At last , obtain the joint probability distribution ( PDF ) between annotation words and testing images using Naive Bayes model finally , then build the annotation model .
最后利用朴素贝叶斯模型来得到标注词与测试图像的联合概率分布,构筑此标注模型。
-
The word sense classifiers established by Naive Bayes model , Maximum Entropy method and Support Vector Machine were used as reference models in experiments which the effectiveness of Bayesian model improved by information gain .
实验中以朴素贝叶斯模型、最大熵方法和支持向量机建立的词义分类器作为参照模型,讨论信息增益改进贝叶斯模型的效果。
-
The performance of the N-gram model classifier was compared with that of the traditional models ( Vector Space Model and Naive Bayes Model ) . Experiment result shows that the accuracy and the stability of the N-gram model classifier are better than others .
通过N元语言模型与传统分类模型(向量空间模型和NaiveBayes模型)的实验对比,结果表明:N元模型分类器具有更好的分类性能。
-
Naive Bayes model is a kind of classifier base on rate statistics , although Naive Bayes model base on the independence assumption , but it 's still a very efficient classifier . Experiment proof it 's categorization veracity can attain 90 % .
贝叶斯方法是一种基于概率统计的分类方法,虽然朴素贝叶斯分类方法是基于特征项之间相互独立的假设,但它依然是一个非常有效的分类器,实验证明它的分类准确度最高可达90%。
-
By the comparative study of some models , The Naive Bayes model and Maximum Entropy are better than other models in performance , especially the Na ? ve Bayes model , it is convenient over construction and realization , and machine learning process is brief and efficient .
通过各个模型的比较研究,发现贝叶斯模型和最大熵模型性能比其它几个模型更为理想,尤其是贝叶斯模型,在构建和实现上比其它几个模型相对要简便易用,机器学习过程也简洁高效。
-
Improving Naive Bayes Classification Model in Various Methods
多种策略改进朴素贝叶斯分类器
-
The basic theory of Bayes classification and the Naive Bayes classification model are introduced . 2 .
本文主要工作如下:1、介绍了贝叶斯分类的基本理论和朴素贝叶斯分类模型。
-
The system adopts supervised learning , Naive Bayes as the categorization model and information gain as the feature selection .
系统采用有指导的学习方法,选取NaiveBayes作分类模型和信息增益作为特征提取方法。
-
Finally , designing the Naive Bayes trust inference algorithm to identify the influent of the comments to the review and mining the influential figures , then build a multilayer Naive Bayes model to detection false topics of micro-blog .
最后,设计朴素贝叶斯的信任推理算法实现对有影响力人物的发现以及识别评论者及其评论文本对被评论文本的影响,最后构建多层朴素贝叶斯模型实现对微博中虚假话题的检测。